Meta Description

Courtesy: Unsplash
Imagine a vibrant dance floor, teeming with data points swirling and twirling. Each data point, like a dancer, holds a story, a piece of information waiting to be revealed. But amidst the captivating chaos, understanding the meaning behind the movement, the rhythm and rhyme of the data, can be daunting. That’s where statistical salsa comes in, a powerful tool for decoding the data dance and uncovering the hidden narratives within.
This journey into data dance begins with understanding the fundamentals of statistics:
Now, let’s delve into the key statistical moves that help you decode the data dance:
- Central Tendency: This is where you find the “average” dancer, the typical point around which the data tends to cluster. Measures like mean, median, and mode offer different perspectives on the centre of the dance floor.
- Dispersion: But not all dancers move the same way. Dispersion measures like variance and standard deviation tell you how spread out the dancers are, revealing the diversity and variability within the data.
- Relationships: The data dance isn’t just about individual steps; it’s about how the dancers interact. Correlation and regression analysis assess how one data point’s movement influences another, uncovering hidden patterns and causal relationships.
- Probability: Sometimes, the data dance gets unpredictable. Probability helps you understand the chances of certain movements occurring, allowing you to make informed decisions based on the inherent uncertainty in the data.
- Hypothesis Testing: This is where you become the choreographer, proposing a specific move or pattern you want to observe in the data. By testing your hypothesis against statistical evidence, you can confirm or reject your predictions, adding clarity and direction to your analysis.
But mastering statistical salsa isn’t just about knowing the steps; it’s about interpretation and storytelling. Here’s where your analytical prowess comes into play:
- Contextualization: Don’t just interpret the data in isolation. Consider the context, the specific research question, and the limitations of the study. This ensures your interpretations are meaningful and relevant to the real world.
- Visualization: Charts and graphs are your visual aids, helping you translate the data dance into a format that resonates with your audience. Choose the right visualization to effectively communicate your findings and engage the reader.
- Avoiding Common Pitfalls: Beware of statistical biases, data manipulation, and overgeneralization. Be transparent about your methods, acknowledge limitations, and let the data guide your interpretations, not the other way around.

Remember, statistical salsa is a collaborative dance. Don’t hesitate to seek help and feedback from peers, mentors, and statisticians. Sharing your analysis and interpretations can lead to richer insights and broaden your understanding of the data dance.
Finally, keep in mind that data analysis is an iterative process. As you delve deeper, you may discover new patterns, uncover new questions, and need to refine your approach. Embrace the dance, be flexible, and most importantly, have fun exploring the stories hidden within the data.
With dedication, practice, and a dash of creativity, you’ll be well on your way to becoming a master of statistical salsa, decoding the data dance and transforming it into meaningful, impactful insights that inform and inspire.
Statistical Salsa: Mastering the Moves with Real-World Examples
Imagine yourself analysing data, not as a passive observer, but as a choreographer guiding the “data dance.” Statistical techniques are your steps, transforming raw information into meaningful insights. Let’s explore some key moves with real-world examples:
1. Central Tendency: Finding the “Typical” Dancer:
- Mean: Imagine studying the weight of students in a class. The mean weight might represent the “average” student, but it can be skewed by outliers like athletes.
- Median: In this case, the median weight might be more representative, as it reflects the middle value, unaffected by extreme values.
- Mode: Analysing clothing sizes in a store inventory, the mode (most frequent size) might be crucial for stocking decisions.
2. Dispersion: Understanding the Spread:
- Variance: Imagine studying test scores. High variance indicates a wide spread, with some students scoring much higher or lower than the average.
- Standard Deviation: This quantifies the spread, helping understand how much individual scores deviate from the average. Analysing customer purchase amounts, a high standard deviation might suggest diverse spending habits requiring different marketing strategies.
3. Relationships: Unveiling Hidden Connections:
- Correlation: Analysing exercise frequency and health outcomes, a positive correlation might suggest that more exercise leads to better health (but not necessarily cause-and-effect).
- Regression: Studying the relationship between advertising spending and sales, regression analysis can estimate the potential impact of increased advertising budget on sales.
4. Hypothesis Testing: Testing Predictions:
- T-test: Comparing the average website traffic before and after a redesign, a t-test can help determine if the redesign actually increased traffic.
- Chi-square test: Analysing the association between smoking and lung cancer, a chi-square test can help assess if there’s a statistically significant relationship.
5. Probability: Predicting the Unpredictable:
- Normal Distribution: Predicting customer service wait times, a normal distribution model can estimate the likelihood of different waiting times.
- Monte Carlo Simulation: Simulating the spread of a disease, a Monte Carlo simulation can estimate the potential impact of different intervention strategies.
Remember, statistical salsa isn’t just about individual moves; it’s about combining them to tell a story. By understanding the fundamentals, choosing the right techniques, and interpreting them in context, you can transform data from a chaotic dance floor into a captivating narrative with real-world impact. So, step onto the floor, master the moves, and let the data dance reveal its hidden stories.

Stepping Beyond the Basics: Exploring Diverse Statistical Approaches
The world of statistics is vast, offering a multitude of approaches beyond the fundamental steps discussed earlier. Let’s delve into some specific examples, drawing on relevant literature to illustrate their applications in various research contexts:
1. Unravelling Complex Relationships:
- Structural Equation Modelling (SEM): Imagine studying the factors influencing student academic performance. SEM allows you to simultaneously analyse the direct and indirect effects of variables like socioeconomic background, study habits, and teacher quality, providing a more nuanced understanding than traditional regression analysis. (e.g., Hair, J. F., et al. (2019). Partial least squares structural equation modelling (PLS-SEM): An updated guide and practical guidelines for sustained growth).
2. Classification and Prediction:
- Machine Learning Techniques: Analysing medical imaging data to identify cancerous tumours, machine learning algorithms like Random Forests can classify images with high accuracy, aiding in diagnosis and treatment decisions. (e.g., Bui, T. D., et al. (2020). A review of deep learning in medical image segmentation and classification).
3. Analyzing Longitudinal Data:
- Mixed-effects Models: Studying the impact of a new educational program on student achievement over time, mixed-effects models account for individual differences and changes across time points, offering a more accurate picture of program effectiveness. (e.g., Snijders, T. A. B., & Bosker, R. J. (2012). Multilevel analysis: An introduction to basic and advanced multilevel modelling).
4. Exploring Hidden Structures:
- Factor Analysis: Analysing survey data on personality traits, factor analysis identifies underlying factors that explain the correlations between different items, offering insights into the structure of personality. (e.g., Fabrigar, L. R., Wegener, D. T., & MacCallum, R. C. (1999). Exploratory factor analysis for ordinal variables: A tutorial).
5. Dealing with Big Data:
- Data Mining Techniques: Analysing massive datasets from social media platforms, data mining techniques like association rule learning can uncover hidden patterns and relationships, revealing user behaviour and trends. (e.g., Han, J., Kamber, M., & Pei, J. (2012). Data mining: Concepts and techniques).
These are just a few examples, and the choice of approach depends on the specific research question, data type, and desired outcome. Consulting relevant literature in your field can guide you toward the most appropriate techniques and provide valuable insights into their applications and limitations.
Remember, statistical salsa isn’t just about knowing the steps; it’s about understanding the music, the context, and the choreography. By drawing on relevant literature, you can expand your repertoire, master diverse moves, and ultimately create a data dance that resonates with your audience and contributes meaningful insights to your field.
Statistical Salsa with Soul: Ethical Considerations for Responsible Data Dance
While mastering the steps of statistical analysis is crucial, remember that the data dance shouldn’t come at the cost of ethical compromises. Just like any dance form, statistical salsa demands responsibility and transparency. Here’s why:
- Respecting Individuality: Data points represent individuals, and their privacy must be protected. Anonymization and informed consent are crucial, especially when dealing with sensitive information. Consider the ethical implications of data collection, storage, and usage at every step. (e.g., General Data Protection Regulation (GDPR) guidelines)
- Avoiding Bias: Algorithmic biases can creep into statistical analysis, potentially leading to discriminatory outcomes. Be mindful of potential data collection, sampling, and model selection biases. Use diverse datasets and transparent methods to mitigate bias and ensure fairness. (e.g., Barocas, S., & Selbst, A. D. (2016). Fairness in machine learning).
- Understanding Context: Refrain from overgeneralizing or misinterpreting findings. Consider the context and limitations of your study before concluding. Avoid misleading visualizations or cherry-picking results to fit a narrative. Be honest about uncertainties and potential alternative explanations. (e.g., American Statistical Association Ethical Guidelines for Statistical Practice)
- Transparency is Key: Document your methodology clearly, including data sources, statistical techniques, and assumptions made. Share your code and data (where possible) to allow for independent verification and reproducibility. Openness encourages trust and allows others to build upon your work. (e.g., Open Science Framework)
- Societal Impact: Consider the potential societal impact of your findings. How might your data dance be used or misused? Be aware of the implications for privacy, social justice, and equality. Advocate for responsible use of data and engage in discussions about potential ethical concerns.
Statistical salsa isn’t just about technical proficiency; it’s about using data analysis for good. By integrating ethical considerations throughout your research journey, you’ll ensure responsible data practices and contribute to a more just and equitable society. Remember, your data dance can tell meaningful stories. Still, these stories should be told with respect, transparency, and a deep sense of responsibility towards the individuals and communities represented by the data points on the dance floor.

Deepening the Groove: Exploring Advanced Statistical Concepts
As you progress on your statistical salsa journey, the dance floor expands, offering intricate moves like machine learning and Bayesian statistics. Let’s delve into these advanced concepts, assuming you have some background knowledge:
- Machine Learning: From Models to Predictions: Imagine building an AI tango partner that learns from your moves and anticipates your next step. Machine learning algorithms, like random forests or support vector machines, analyse vast datasets, identify patterns, and make predictions. This can be used for tasks like image recognition, spam filtering, or predicting customer behaviour. But remember, these models are only as good as the data they’re trained on, and ethical considerations like bias and explainability remain crucial.
- Bayesian Statistics: Embracing Uncertainty: Unlike traditional frequentist statistics focusing on “true” population parameters, Bayesian statistics embraces uncertainty. It incorporates prior knowledge and updates beliefs as new data arrives, offering flexibility and dynamic interpretation. Think of it like a tango partner adapting to your changing lead, constantly refining its understanding based on your moves. This approach is precious in fields like medical research, where data and uncertainty are often scarce.
- Dimensionality Reduction: Conquering the Data Jungle: Imagine navigating a dense rainforest of variables. Dimensionality reduction techniques, like Principal Component Analysis, help condense complex datasets into manageable representations, preserving key information while making analysis easier. Think of it like clearing a path through the jungle, revealing the underlying structure of the data. This is crucial for analysing high-dimensional datasets in fields like genomics or finance.
- Time Series Analysis: Dancing Through Time: Imagine analysing the rhythm of stock prices or the tempo of heartbeats. Time series analysis delves into data points collected over time, identifying trends, seasonality, and potential forecasting opportunities. Think of it like adapting your tango steps to the changing melody of the music, anticipating the next beat. This technique is invaluable for understanding dynamic phenomena in econometrics or climate science fields.
Remember, these are just a few advanced moves in the statistical salsa repertoire. As you explore further, consult relevant literature, engage with experts, and practice applying these concepts to your specific research questions. By mastering these advanced steps, you’ll be well-equipped to navigate the complexities of the data dance floor, extracting deeper insights and contributing to ground-breaking discoveries in your field.
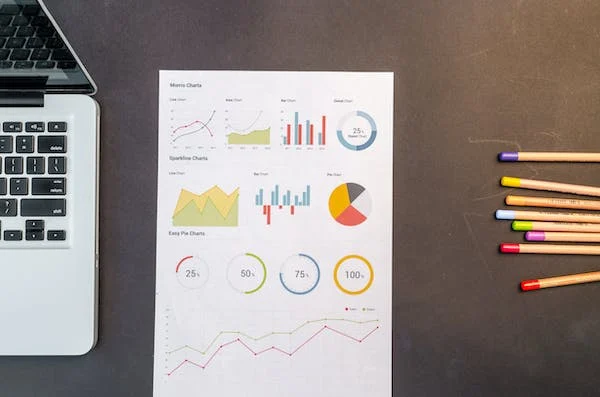
Conclusion
Statistical salsa isn’t just an academic pursuit; it’s a key skill for navigating our data-driven world. By embracing its potential, you can unlock valuable insights from various fields, from social sciences to healthcare, business, and beyond.
So, step onto the data dance floor, embrace the rhythm, and let your curiosity guide you. With each move you master, you become a powerful storyteller, transforming data into insights that shape a better future. The world awaits your unique choreography, so put on your dancing shoes and get ready to unlock the stories hidden within the data.